Note
Click here to download the full example code
Feature Extraction for Denoising: Clean and Noisy Audio¶
Extract acoustic features from clean and noisy datasets for training a denoising model, e.g. a denoising autoencoder.
To see how soundpy implements this, see soundpy.builtin.denoiser_feats
.
import os, sys
import inspect
currentdir = os.path.dirname(os.path.abspath(
inspect.getfile(inspect.currentframe())))
parentdir = os.path.dirname(currentdir)
parparentdir = os.path.dirname(parentdir)
packagedir = os.path.dirname(parparentdir)
sys.path.insert(0, packagedir)
import soundpy as sp
import IPython.display as ipd
package_dir = '../../../'
os.chdir(package_dir)
sp_dir = package_dir
Prepare for Extraction: Data Organization¶
I will use a mini denoising dataset as an example
# Example noisy data:
data_noisy_dir = '{}../mini-audio-datasets/denoise/noisy'.format(sp_dir)
# Example clean data:
data_clean_dir = '{}../mini-audio-datasets/denoise/clean'.format(sp_dir)
# Where to save extracted features:
data_features_dir = './audiodata/example_feats_models/denoiser/'
Choose Feature Type¶
We can extract ‘mfcc’, ‘fbank’, ‘powspec’, and ‘stft’. if you are working with speech, I suggest ‘fbank’, ‘powspec’, or ‘stft’.
feature_type = 'stft'
sr = 22050
Set Duration of Audio¶
How much audio in seconds used from each audio file. the speech samples are about 3 seconds long.
dur_sec = 3
Option 1: Built-In Functionality: soundpy does everything for you¶
Define which data to use and which features to extract. NOTE: beacuse of the very small dataset, will set perc_train to a lower level than 0.8. (Otherwise, will raise error) Everything else is based on defaults. A feature folder with the feature data will be created in the current working directory. (Although, you can set this under the parameter data_features_dir) visualize saves periodic images of the features extracted. This is useful if you want to know what’s going on during the process.
perc_train = 0.6 # with larger datasets this would be around 0.8
extraction_dir = sp.denoiser_feats(
data_clean_dir = data_clean_dir,
data_noisy_dir = data_noisy_dir,
sr = sr,
feature_type = feature_type,
dur_sec = dur_sec,
perc_train = perc_train,
visualize=True);
extraction_dir
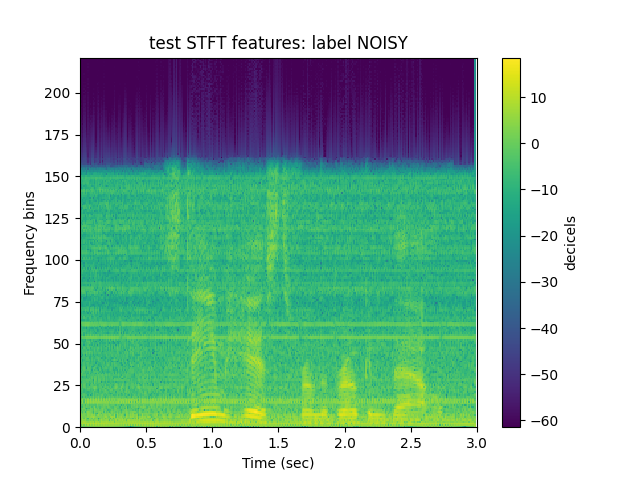
Out:
/home/airos/Projects/github/a-n-rose/Python-Sound-Tool/soundpy/files.py:352: UserWarning: Some files did not match those acceptable by this program. (i.e. non-audio files) The number of files not included: 3
warnings.warn(message)
/home/airos/Projects/github/a-n-rose/Python-Sound-Tool/soundpy/feats.py:2383: UserWarning:
WARNING: `win_size_ms` was not set. Setting it to 20 ms
warnings.warn(msg)
/home/airos/Projects/github/a-n-rose/Python-Sound-Tool/soundpy/feats.py:2390: UserWarning:
WARNING: `percent_overlap` was not set. Setting it to 0.5
warnings.warn(msg)
16% through train stft feature extraction
33% through train stft feature extraction
50% through train stft feature extraction
66% through train stft feature extraction
83% through train stft feature extraction
100% through train stft feature extraction
Features saved at audiodata/example_feats_models/denoiser/features_9m3d13h26m1s569ms/train_data_clean.npy
50% through val stft feature extraction
100% through val stft feature extraction
Features saved at audiodata/example_feats_models/denoiser/features_9m3d13h26m1s569ms/val_data_clean.npy
50% through test stft feature extraction
100% through test stft feature extraction
Features saved at audiodata/example_feats_models/denoiser/features_9m3d13h26m1s569ms/test_data_clean.npy
16% through train stft feature extraction
33% through train stft feature extraction
50% through train stft feature extraction
66% through train stft feature extraction
83% through train stft feature extraction
100% through train stft feature extraction
Features saved at audiodata/example_feats_models/denoiser/features_9m3d13h26m1s569ms/train_data_noisy.npy
50% through val stft feature extraction
100% through val stft feature extraction
Features saved at audiodata/example_feats_models/denoiser/features_9m3d13h26m1s569ms/val_data_noisy.npy
50% through test stft feature extraction
100% through test stft feature extraction
Features saved at audiodata/example_feats_models/denoiser/features_9m3d13h26m1s569ms/test_data_noisy.npy
Finished! Total duration: 2.32 seconds.
PosixPath('audiodata/example_feats_models/denoiser/features_9m3d13h26m1s569ms')
The extracted features, extraction settings applied, and which audio files were assigned to which datasets will be saved in the extraction_dir directory
Logged Information¶
Let’s have a look at the files in the extraction_dir. The files ending with .npy extension contain the feature data; the .csv files contain logged information.
featfiles = list(extraction_dir.glob('*.*'))
for f in featfiles:
print(f.name)
Out:
val_data_noisy.npy
dataset_audio_assignments.csv
val_data_clean.npy
test_data_noisy.npy
audiofiles_datasets_clean.csv
test_data_clean.npy
log_extraction_settings.csv
train_data_clean.npy
clean_audio.csv
noisy_audio.csv
train_data_noisy.npy
audiofiles_datasets_noisy.csv
Feature Settings¶
Since much was conducted behind the scenes, it’s nice to know how the features were extracted, for example, the sample rate and number of frequency bins applied, etc.
feat_settings = sp.utils.load_dict(
extraction_dir.joinpath('log_extraction_settings.csv'))
for key, value in feat_settings.items():
print(key, ' ---> ', value)
Out:
dataset_dirs ---> ['../../../../mini-audio-datasets/denoise/noisy', '../../../../mini-audio-datasets/denoise/noisy', '../../../../mini-audio-datasets/denoise/noisy']
feat_base_shape ---> (299, 221)
feat_model_shape ---> (299, 221)
complex_vals ---> True
context_window --->
frames_per_sample --->
labeled_data ---> False
decode_dict --->
visualize ---> True
vis_every_n_frames ---> 50
subsection_data ---> False
divide_factor ---> 5
total_audiofiles ---> 10
kwargs ---> {'sr': 22050, 'feature_type': 'stft', 'dur_sec': 3, 'win_size_ms': 20, 'percent_overlap': 0.5}
Total running time of the script: ( 0 minutes 2.400 seconds)